What the "Age of Alpha-Fold" means for Biochemistry
- Grant Wiersum
- Jun 22, 2022
- 4 min read
1 - An Introduction to Computational Structure Determination
While artificial intelligence (AI) implementation in pharmaceuticals development lags behind many consumer industries, there are a number of events that may be potential signs of a paradigm-shift. Every cellular process, whether beneficial or malign, is enacted and mediated by the actions of proteins. Every protein is built from a sequence of just 20 amino-acids. As such, it can be relatively simple to code protein sequences into a form that computers can easily handle. What has been much more difficult, however, is building models that can make accurate predictions based on that sequence. This challenge is often known as the “folding problem” ; few insights can be drawn into a protein’s function based on a sequence. What needs to be known is how that sequence is arranged in 3D space. Knowing a proteins structure allows us to build a map of its surface, its energetic topography and build models that let us predicts what compounds are likely to augment or hinder its activity.
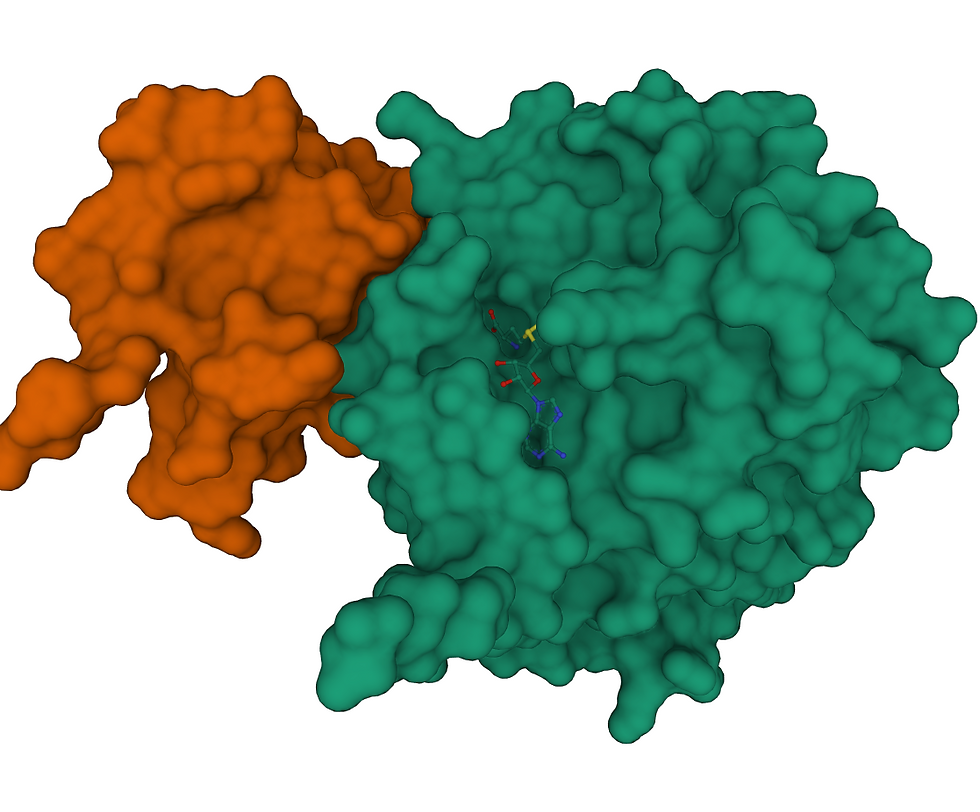
To meet the folding problem, comes AlphaFold. Perhaps the greatest single breakthrough for the area of protein-biochemistry in the last century came in 2018 with DeepMind’s announcement of AlphaFold and AlphaFold2. Previously, proteins had to be identified, cloned, grown, purified and either crystallized, or analyzed by nuclear-magnetic-resonance (NMR) or cryo-electron-microscopy. Each step in the structural-biology chain is extremely expensive, both financially and in hours of work, and comes with its own rate of failure. What can be accomplished using traditional methods over a decade of work, AlfaFold can often approximate in just a few days (Jumper et al. 2021). With the crisis posed by SARS-CoV2, came an opportunity: To meet the challenge and demonstrate the usefulness of their model, the AlphaFold team fed the genome of the SARS-CoV2 virus to their model. The result was the publication of six structures for proteins they determined likely to give researchers the most trouble through traditional methods. Through the structures and methods published by the AlphaFold team, researchers have been able to accelerate their research, generating better structural models more quickly with fewer resources (Heo, Janson and Feig 2021 ; Muller 2021). Because of the degree of difficulty posed by the folding problem, predicted structures still have a large degree of uncertainty where enormousis precision is required. However, the true utility AlfaFold provides is in guiding research efforts, providing a kind of high-level map of where to direct time and money.
2- An Atomic View
Neural-network-based at its core, the underlying algorithm of AlphaFold starts fairly inauspiciously. Multiple-sequence-alignment (MSA) is possibly the technique underpinning the entire field of bioinformatics and a surprisingly conventional place to start. From MSA, a family tree of proteins is built from homologous proteins, whose structures are already known. This MSA matrix then undergoes a series of transformations with the goal of reducing dimensionality and computational overhead. The generated matrix now consists of amino-acid sequences aligned side-by-side, paired with their structures, and represented by the locations of their individual atoms in 3-D space. The encoded spatial relationships then undergo “triangle-multiplication” whereby amino-acids are selected in trios and the model learns from their relationships in space, relative to one another. The model iterates like this, tuning the weights it assigns to individual atoms and their behaviors within the protein backbone. Because of the use of multiple alignments, the model has the added benefit of learning within the confines of evolutionary possibility. Finally, a test can be administered, using a protein the model has not seen yet. Total error can be calculated by the mean distance between each pair of predicted versus actual atomic positions.

The training dataset was scraped from the existing body of work hosted by the Protein DataBank (PDB). Additional data was generated artificially by using a “noisy student” paradigm under which one model is trained on only available data. This “teacher” model then generates a new dataset which can be used to augment the available data (Jumper 2021) Models trained under this paradigm have been seen to generalize better than the “teacher” model in several cases (Xie, Luong, Hovy and Le 2020).
3 - Reflections on Meta-Cognition
The real lessons to be learned from AlphaFold come from its incredible application of existing methods, augmented by judicious application of a neural-network architecture. There can be a tendency on the part of those with only a passing familiarity with neural-networks to view them as a black-box into which training data is fed and a predictive model comes out. AlpaFold demonstrates the importance of a thorough understanding of the available methods and architectures, coupled with a deep domain knowledge. By using established methods for generating bioinformatic insight to generate a matrix that can be fed to existing algorithms for semantic image segmentation (Huang et al. 2019) and recognition. The real edge generated by this level of focus is the relatively low computational cost of training AlphaFold as compared to other models (Jumper et al. 2020).
This takeaway leads one to wonder: at what point can even the task of architecture development be automated - if ever? We currently see tools like GitHub’s copilot gaining adoption and usefulness. It wouldn’t be unreasonable to imagine a model, trained on the entirety of code publicly available (which is truly immense), generating machine-learning architectures current researchers are incapable of. When AlphaFold is surpassed by more than mere incremental gains, it will undoubtedly be because an architecture was developed with the stated purpose of building better architectures, ushering in an era of general-purpose AI.
References
Heo, L, Janson, G, Feig, M. 2021. Physics-based protein structure refinement in the era of artificial intelligence. Proteins. 89( 12): 1870- 1887. https://doi.org/10.1002/prot.26161
Z. Huang, X. Wang, L. Huang, C. Huang, Y. Wei and W. Liu, 2019 CCNet: Criss-Cross Attention for Semantic Segmentation, IEEE/CVF International Conference on Computer Vision (ICCV), 2019, pp. 603-612, doi: 10.1109/ICCV.2019.00069.
Jumper, J., Evans, R., Pritzel, A. et al. 2021. Highly accurate protein structure prediction with AlphaFold. Nature 596, 583–589 . https://doi.org/10.1038/s41586-021-03819-2
Mullard, A. 2021, What does AlphaFold mean for drug discovery?, Nature, https://www.nature.com/articles/d41573-021-00161-0
Savage, N., 2021, Tapping into the drug discovery potential of AI, Nature,
Xie, Q., Luong, M., Hovy, E., Quoc, L.V., 2020, Self-Training with Noisy Student Improves ImageNet Classification, Proceedings of the IEEE/CVF Conference on Computer Vision and Pattern Recognition, pp.10687-10698. https://openaccess.thecvf.com/content_CVPR_2020/html/Xie_Self-Training_With_Noisy_Student_Improves_ImageNet_Classification_CVPR_2020_paper.html
Commenti